If you want to open and grow your business, you first have to check if your product or service is market fit or not. We also did the same with this blog. But do you know that we failed FOUR times before it reached you?
But what is the reason? It’s not that we didn’t know data cleaning and how to implement it to improve the quality of the data analysis. Then what is it? Can you guess it?
After failing to gain traction with our blog for the 4th consecutive time, then one of our low-grade researchers told us this, “I think people don’t know why to implement data cleaning, that’s why maybe they are not reading this”. We were shocked by that answer. Then in the 5th time when we incorporated this point in our blog, then all the readers got engaged in our research-backed blog.
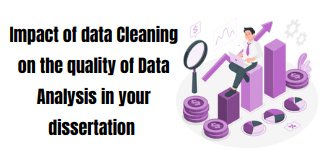
In this blog, we are not only going to talk about the impact of data cleaning to improve the quality of your data analysis but also we are going to save a lot of your precious time so that you don’t face this problem again.
This blog has been divided into 4 parts and more. To understand the other portions of the blog, you need to study the initial 4 parts. So, let’s get started 🙇.
How to Analyze data in research
The process of analysing data in research involves several steps. Here is a general overview of the steps involved in analyzing data in research:
- Prepare the data: This involves organizing and cleaning the data, which includes checking for missing values, removing outliers, and transforming the data into a format suitable for analysis.
- Explore the data: This step involves examining the data using descriptive statistics and visualizations to identify patterns, trends, and relationships in the data. This can be done using techniques such as histograms, scatterplots, and correlation matrices.
- Formulate research questions: Based on the exploration of the data, research questions can be formulated that address the patterns, trends, and relationships identified in the data.
- Select the appropriate statistical methods: Based on the research questions, appropriate statistical methods can be selected to analyze the data. This can include techniques such as t-tests, ANOVA, regression analysis, and factor analysis.
- Conduct statistical analyses: The selected statistical methods are applied to the data to test the research questions and hypotheses.
- Interpret the results: The results of the statistical analyses are interpreted in the context of the research questions and hypotheses, and conclusions are drawn based on the findings.
- Communicate the findings: Finally, the findings are communicated in a clear and concise manner using tables, graphs, and other visual aids to facilitate understanding by the intended audience.
Overall, data analysis in dissertation involves careful preparation, exploration, and interpretation of the data and appropriate statistical methods to address the research questions and hypotheses.
Important steps in Data analysis
Data analysis is a process of examining and interpreting data using statistical and analytical methods to extract meaningful insights and make informed decisions. Here are some important steps in data analysis:
- Define the research question: The first step in data analysis is to define the research question or problem that needs to be addressed. This helps in identifying the relevant data sources and the analytical methods required for the analysis.
- Collect the data: The next step is to collect the data from various sources such as surveys, databases, or websites. The data should be relevant, accurate, and reliable.
- Clean the data: Data cleaning involves identifying and correcting errors, removing missing values, and outliers, and transforming the data into a format suitable for analysis.
- Explore the data: Data exploration involves summarizing the data using descriptive statistics and visualizations to identify patterns, trends, and relationships in the data.
- Analyze the data: Data analysis involves applying appropriate statistical and analytical methods to the data to answer the research question or problem. This can include techniques such as regression analysis, hypothesis testing, and machine learning.
- Interpret the results: The results of the data analysis are interpreted to draw conclusions and make informed decisions. This involves assessing the statistical significance of the findings and their practical implications.
- Communicate the findings: The final step is to communicate the findings to the stakeholders in a clear and concise manner using visualizations, reports, or presentations. This helps in ensuring that the insights are understood and acted upon.
Overall, data analysis involves a systematic and rigorous approach to examining and interpreting data to extract insights and make informed decisions. It is a critical step in data-driven decision-making and requires a combination of technical expertise, domain knowledge, and analytical skills.
A Step-by-Step Guide to the Data Analysis Process
Apart from the steps given above, there are also some steps which are essential for conducting data analysis such as:
- Pre-processing the data: Pre-processing involves transforming the data to prepare it for modelling. This can include feature engineering, scaling, and normalization.
- Build the model: The next step is to select and build a statistical or machine-learning model to analyze the data. This involves selecting an appropriate algorithm, optimizing the model parameters, and training the model on the data.
- Evaluate the model: The model is evaluated to assess its performance using metrics such as accuracy, precision, recall, and F1 score. This helps in identifying any issues with the model and refining it to improve its performance.
Now, another important question comes into play which is what are the things required to keep in mind while conducting data analysis? Here are some important things to keep in mind while conducting data analysis:
- Understand the context: Before analyzing the data, it’s important to understand the context in which the data was collected. This includes understanding the research question, the data collection methods, and the limitations of the data.
- Use appropriate techniques: It’s important to use appropriate analytical techniques to analyze the data. This involves selecting the right statistical tests, models, or algorithms based on the research question and the type of data.
- Validate the data: It’s important to validate the data before analyzing it to ensure that it’s accurate and reliable. This involves checking for errors, missing values, and outliers and verifying the data with external sources if possible.
- Consider ethical implications: It’s essential to consider the ethical implications of the data analysis, such as data privacy, confidentiality, and bias. This involves following ethical guidelines and ensuring that the study is conducted in a responsible and transparent manner.
Data analysis requires careful planning, attention to detail, and ethical considerations. By keeping these things in mind, you can ensure that your data analysis is accurate, reliable, and actionable.
Tools used in Data cleaning
There are several tools used in data cleaning to identify errors, inconsistencies, and missing values in the data. Here are some commonly used data-cleaning tools:
- OpenRefine: OpenRefine is a free, open-source tool used for cleaning and transforming data. It can handle large datasets and has features for cleaning and standardizing data, removing duplicates, and identifying outliers.
- Excel: Excel is a popular tool for data cleaning and analysis. It has built-in features for sorting, filtering, and formatting data, and can be used to detect and remove errors and inconsistencies.
- Trifacta: Trifacta is a commercial data preparation tool that helps users to clean, transform, and integrate data. It has a user-friendly interface and uses machine learning algorithms to suggest data cleaning and transformation steps.
- Talend: Talend is a popular open-source data integration tool that has features for data cleaning, transformation, and validation. It supports multiple data sources and can be used to automate the data-cleaning process.
- DataWrangler: DataWrangler is a free, web-based tool that helps users to clean and transform data. It has features for removing duplicates, merging columns, and identifying missing values.
Overall, the choice of data cleaning tool depends on the complexity of the data, the size of the dataset, and the specific requirements of the data cleaning process. It’s important to select a tool that is appropriate for the data cleaning task and that can handle the data efficiently and accurately.
Now, the 4 parts have been answered, but it’s not over yet. The reason is that the main question of this blog hasn’t been answered yet. Now, as you have gained a lot of knowledge about data analysis, you can now easily understand the main question.
Data cleaning is a critical step in the data analysis process that has a significant impact on the quality of the data analysis in your dissertation. Here are some ways in which data cleaning affects the quality of data analysis:
- Accuracy of analysis: Data cleaning helps to ensure that the data is accurate, complete, and consistent. This improves the accuracy of the analysis and ensures that the results are reliable and valid.
- Reduction of errors and biases: Data cleaning helps to identify and remove errors and biases in the data, such as missing values, duplicate records, and outliers. This helps to improve the quality of the data and reduces the risk of making incorrect conclusions.
- Improving statistical power: Data cleaning can help to improve the statistical power of the analysis by reducing noise and increasing the signal-to-noise ratio. Results may be more precise and significant as a result.
- Improving efficiency: Data cleaning can improve the efficiency of the data analysis process by reducing the amount of time and effort required to clean and prepare the data. This allows more time for the actual analysis and interpretation of the data.
- Improving data interpretation: Data cleaning can help to improve the interpretation of the data by making it easier to understand and analyze. This can lead to better insights and conclusions.
Overall, data cleaning is a critical step in the data analysis process that has a significant impact on the quality of the data analysis in your dissertation. It helps to ensure that the data is accurate, reliable, and consistent, which is essential for making meaningful conclusions and recommendations.
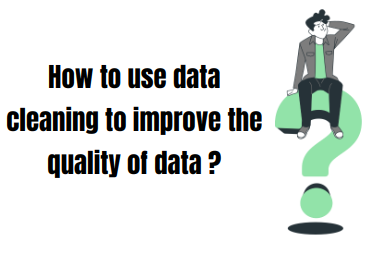
Now, let us know the answer to this question, how to use data cleaning using STATA to improve the quality of the data analysis? So, let us jump into this question.
Data cleaning is an essential step in improving the quality of data analysis. Here are some ways to use data cleaning to improve the quality of data analysis:
- Identify and remove outliers: Outliers can skew the results of the analysis and affect the accuracy of the conclusions. Identifying and removing outliers can help to improve the quality of the data analysis.
- Handle missing values: Missing values can also affect the accuracy of the analysis. Imputing missing values or removing the observations with missing values can help to improve the quality of the data analysis.
- Standardize variables: Standardizing variables can help to make the data more comparable and improve the quality of the analysis. This involves transforming variables to have a mean of zero and standard deviation of one.
- Detect and remove duplicates: Duplicates can also affect the accuracy of the analysis. Detecting and removing duplicates can help to improve the quality of the data analysis.
- Correct data entry errors: Data entry errors can also affect the accuracy of the analysis. Correcting data entry errors can help to improve the quality of the data analysis.
- Check for data consistency: Checking for data consistency, such as making sure that variables are entered consistently across the dataset, can also improve the quality of the analysis.
Overall, data cleaning is an essential step in improving the quality of data analysis. By identifying and removing outliers, handling missing values, standardizing variables, detecting and removing duplicates, correcting data entry errors, and checking for data consistency, you can ensure that the data is accurate, reliable, and consistent, which is essential for making meaningful conclusions and recommendations.
“I want to REALLY know you.” Okay, if you have this in your mind, then please click on this link http://www.geoffandfrancis.co.uk/data-analysis-help.php visit our website to see how we help our students to analyse data at an affordable cost.
If you want to know more about us, you can also check the other services available on our website.
Thank you so much for reading this blog.
Leave a Reply